What is Covariate in Statistics?
What is Covariate in Statistics?
In statistics, a covariate is like an independent variable, which means it is complementary or related to the dependent variable. Any measurable variable that has a statistical relationship with the dependent variable qualifies as a covariate. Therefore, a covariate is a possible explanatory or predictive variable of the dependent variable.
Example of Covariate in Experiment
In an experiment to determine the effect of number of hours studying for a test have on the level of education of the study’s participants, the number of hours the student studied is a covariate. This is the covariate because changes in the number of hours studied correlate with a change in the score the student received on the test.
Use of Covariates in Research
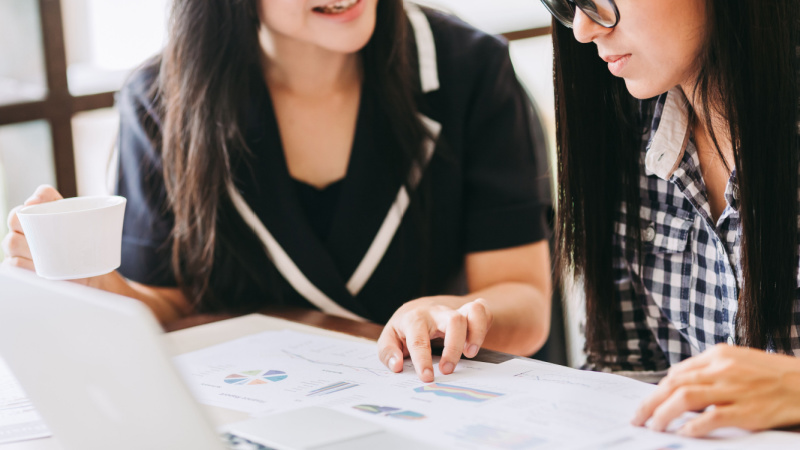
- There are two types of covariate variables, independent variables and confounding variables.
- Independent variables are the ones that are directly being studied.
- Confounding variables are those covariates that are hidden and were not considered by the researcher. You can address and minimize confounding in the design of the study with randomization. When you randomize the study’s participants, confounding variables can be distributed evenly across the comparison groups. Therefore, it is less likely that differences across the comparison will be affected.
Covariates are used in research studies when the observational or experimental units are heterogeneous. In these types of studies, covariates are the X variables in the statistical model, which increase the treatment effects’ precision.
In studies that have observational designs, covariates can be added to investigate specific conditional effects, increase predictive ability, and eliminate confounding.
When to Include a Covariate
- You should include a covariate in your experimental data analysis if the covariate is specified as a priori, such as a pre-analysis plan.
- If you choose a covariate ahead of your experiment and analyze the data with only the covariate included, you can avoid a Type I error inflation (a false positive conclusion) and still have the power-boosting benefits of a covariate.
- Your decision to include covariates need to be theoretically driven. You will need to identify important covariates based on your previous research on the topic you are studying. Doing this is especially important when you have nonexperimental designs because you do not have the benefit of random assignment that will help you mitigate the covariates’ effects.
Not having important covariates can lead to misleading results and cause you to draw incorrect conclusions from the data. However, if you include too many covariates, it can reduce the power of the data analyses to find significant associations between the predictor variables and the outcome variable.
Covariates and Analysis of Covariance (ANCOVA)
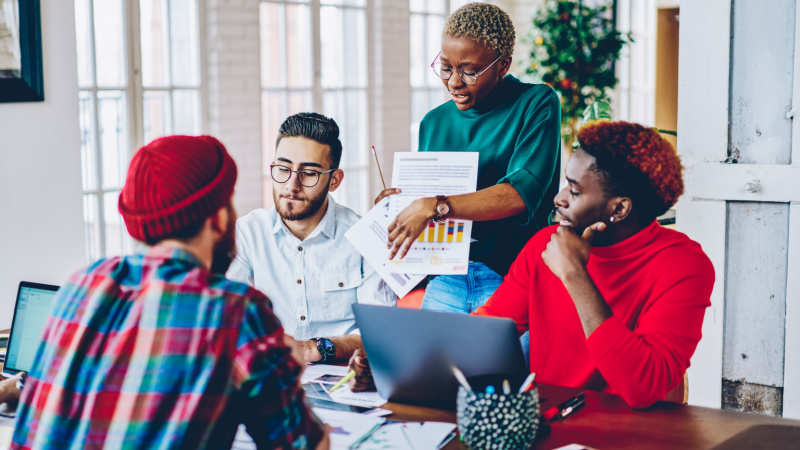
Covariates are often used in experimental designs to account for the influence of an outside variable that may affect the experiment’s results. In experiments in which there are tests of variables, covariates are used as independent control variables.
An analysis of variance (ANOVA) is a statistical test that is used to determine whether there is a statistically significant difference between two or more categorical groups. This is done by testing for differences of means using variance. If you do not have any covariates in your research, then instead of using ANOVA, you need to use analysis of covariance (ANCOVA).
The ANCOVA test includes a continuous variable in addition to the dependent and independent variables as means for control. Here are some of the properties and advantages of ANCOVA:
- ANCOVA ensures homogeneity of regression, which means that it is assumed that the association between the outcome variable and the covariate is similar for each of the groups being tested in the experiment.
- ANCOVA combines analysis of variance and regression analysis to control for the effects of the covariate. It controls the covariates’ effect, thus, enhancing the experiment’s correctness.
- You can use the ANCOVA test in an experimental design to control for factors that can be measured on an interval scale but cannot be randomized.
- You can use it in observational designs to remove the effects of variables that modify the relationship of the independent categories to the interval dependent.
- You can use ANCOVA in non-experimental research, such as non-random samples, surveys, quasi-experiments when participants cannot be randomly assigned to experimental and control groups.
- You can use the one-way ANCOVA to determine whether there are any significant differences between two or more independent (unrelated) groups on a dependent variable. The one-way ANCOVA allows you to statistically control for a third variable, which is commonly known as a confounding variable that will affect your results. This third variable that can confound your results is the covariate.
- If you have two independent variables, you can use a two-way ANCOVA. In this design, you will be determining whether you find a significant two-way interaction effect that you should interpret and any post hoc tests you want to run to learn more about the study’s results.
Source: Statistical Soup: ANOVA, ANCOVA, MANOVA, & MANCOVA
Note: You can refer to the following webpages for more information:
- Understanding Covariates at https://support.minitab.com/en-us/minitab/20/help-and-how-to/statistical-modeling/anova/supporting-topics/anova-models/understanding-covariates/
- What is a Covariate in Statistics? at https://www.statology.org/covariate/